We have all heard of the Hamming Weight of a binary number. Hamming weight is the number of set bits/1s in a binary number. In this problem Number Of 1 bits we have to find the hamming weight of the given number.
Examples
Number = 3
Binary representation = 011
Hamming weight = 2
Number = 4
Binary representation = 100
Hamming weight = 1
A few image representations are gonna help out more
Now, let us look into how to find it out
Table of Contents
Approach – 1A
Brute Force
The Brute force approach would be
- Find n%2 to give us the bit at that position
- Calculate n=n/2 to move to the next bit
Example
Number=5
Let us look at the code.
JAVA Program
class Solution { public: int hammingWeight(uint32_t n) { int ans=0; while(n!=0) { ans=ans+(n%2); n=n/2; } return ans; } };
C++ Program
public class Solution { // you need to treat n as an unsigned value public int hammingWeight(int n) { int ans=0; while(n!=0) { ans=ans+(n%2); n=n/2; } return and; } }
Time complexity = O(n)
Approach – 1B
Brute Force BUT Smarter!
Well, now that we have a simple approach. Let us look more in terms of Bit-Manipulation
First strengthening our game with a few smart points
- We know that an accepted integer can be maximum 32 bits long
- a&1=1 1&0=0
Keeping these things we can loop over 32 and perform an AND operation to find the set bits
JAVA Program for Number Of 1 bits
public class Solution { // you need to treat n as an unsigned value public int hammingWeight(int n) { int ans=0; for(int i=0;i<32;i++) { ans=ans+(n&1); n=n>>1; } return ans; } }
C++ Program for Number Of 1 bits
class Solution { public: int hammingWeight(uint32_t n) { int ans=0; for(int i=0;i<32;i++) { ans=ans+(n&1); n=n>>1; } return ans; } };
Time complexity = O(n)
A lot of approaches we have collected until now are linear. We should surely have in our kitty one smart thing or two to optimize our game. So, looking across I hit upon something gold.
Approach – 2
Brian Kernighan Algorithm
Before getting all erudite and algorithmic let me get you through the process
- We calculate n-1
- We and it with n i.e n&(n-1)
- Thus unset the rightmost bit
- Keep repeating the above steps until we end up with 0
Let number=5
Binary representation=101
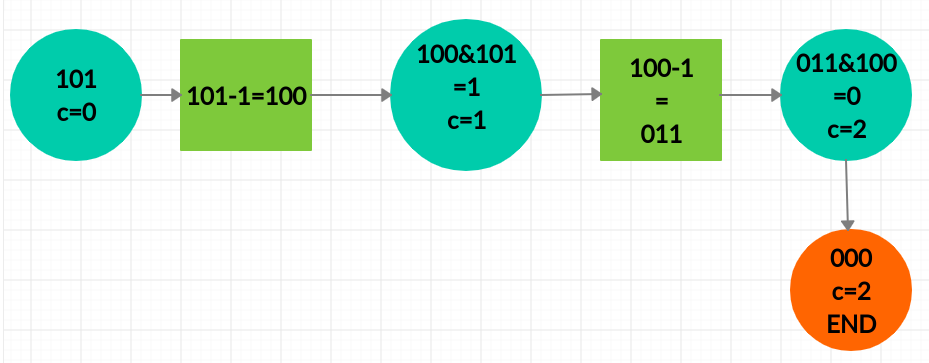
Optimizations
- The algorithm goes through as many iterations as the set bits
Looking into the code
Java Program for Number Of 1 bits
public class Solution { // you need to treat n as an unsigned value public int hammingWeight(int n) { int ans=0; while(n!=0) { n=n&(n-1); ans++; } return ans; } }
C++ Program for Number Of 1 bits
class Solution { public: int hammingWeight(uint32_t n) { int ans=0; while(n!=0) { n=n&(n-1); ans++; } return ans; } };
Analyzing the time complexity
Each integer has log(n) bits and in the worst case we pass through all the bits
Time complexity = Log(n)
Now that we are speeding up how can we miss an approach that takes O(1)
Introducing
Approach – 3
Using Advanced Operations
Let us assume we have a binary number=11010011
We use bitmasks here namely 0101,0011,00001111,00000000011111111 and 000000000000000001111111111111111 to perform the procedure shown in the picture.
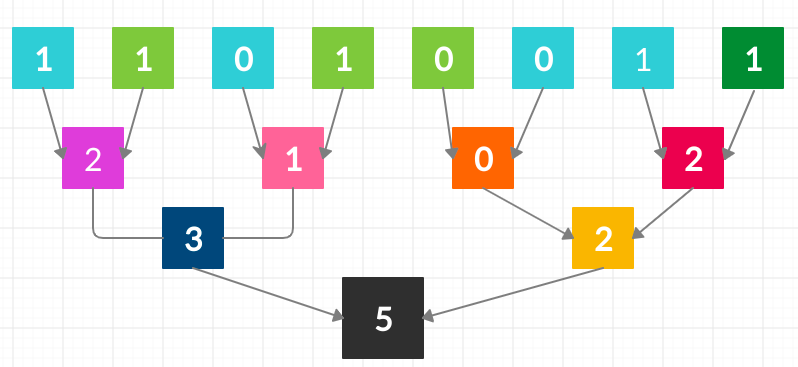
JAVA Program for Number Of 1 bits
public class Solution { // you need to treat n as an unsigned value public int hammingWeight(int n) { n = (n & 0x55555555) + (n >> 1 & 0x55555555); // put count of each 2 bits into those 2 bits n = (n & 0x33333333) + (n >> 2 & 0x33333333); // put count of each 4 bits into those 4 bits n = (n & 0x0F0F0F0F) + (n >> 4 & 0x0F0F0F0F); // put count of each 8 bits into those 8 bits n = (n & 0x00FF00FF) + (n >> 8 & 0x00FF00FF); // put count of each 16 bits into those 16 bits n = (n & 0x0000FFFF) + (n >> 16 & 0x0000FFFF); // put count of each 32 bits into those 32 bits return n; } }
The time complexity of the above approach and the space complexity both end up as O(1).
Wanna know more than just Binary. Tune in!